AI Engineers Must Open Their Designs to Democratic Control
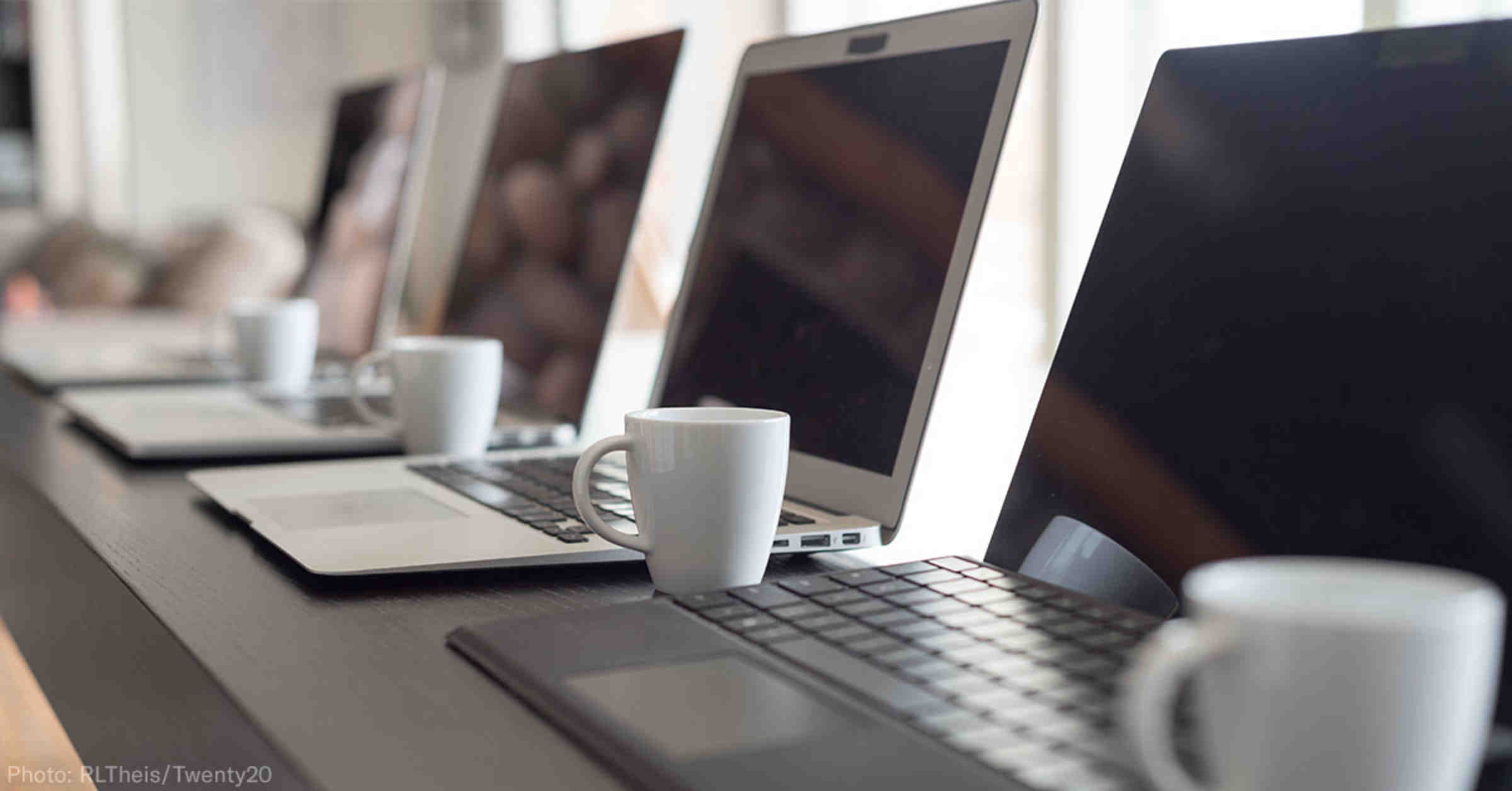
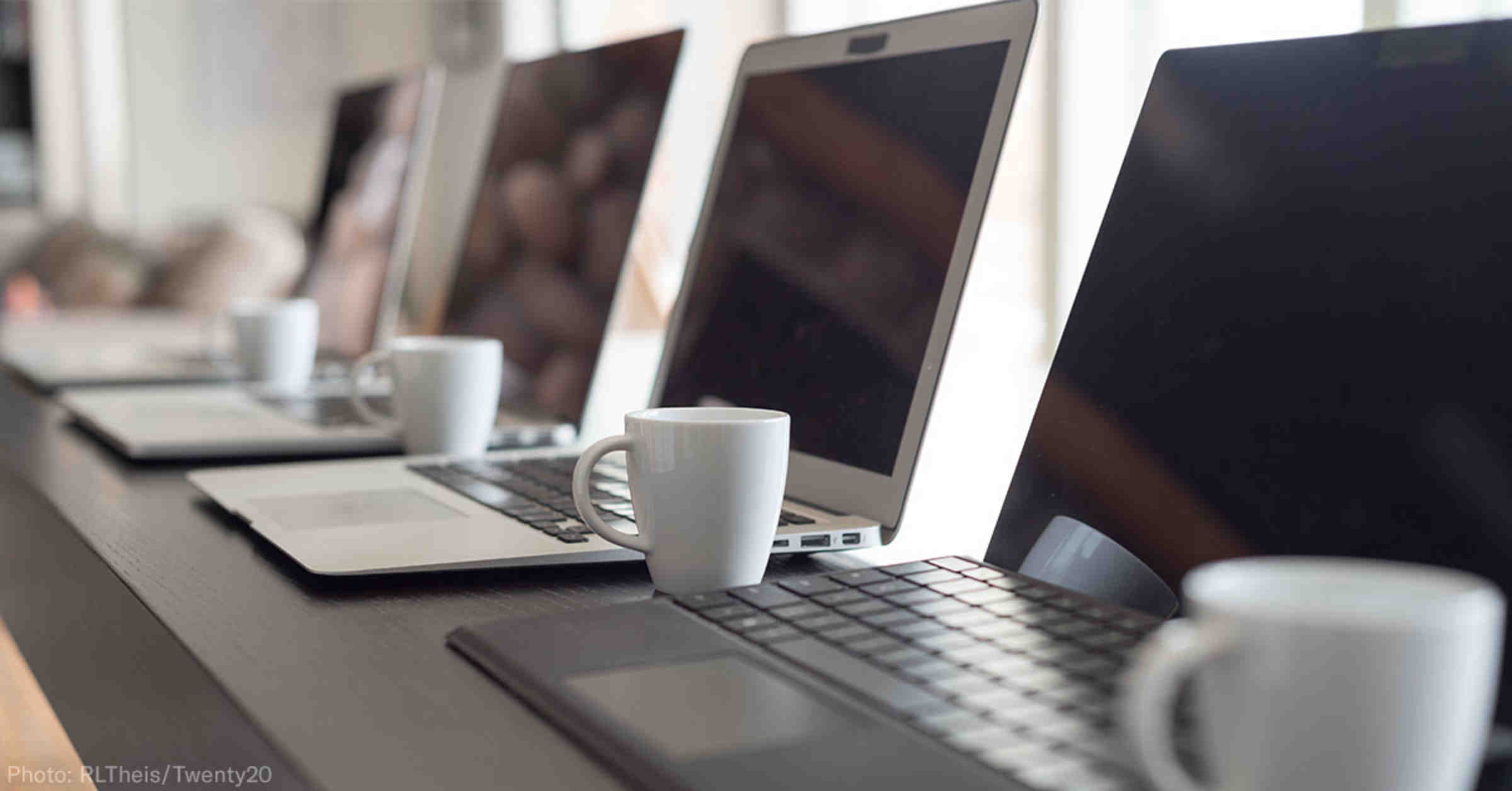
In many ways, the most pressing issues of society today — increasing income disparity, chronic health problems, and climate change — are the result of the dramatic gains in higher productivity we’ve achieved with technology and science. The internet, artificial intelligence, genetic engineering, crypto-currencies, and other technologies are providing us with ever more tools to change the world around us.
But there is a cost.
We’re now awakening to the implications that many of these technologies have for individuals and society. We can directly see, for instance, the effect artificial intelligence and the use of algorithms have on our lives, whether through the phones in our pockets or Alexa on our coffee table. AI is now making decisions for judges about the risks that someone accused of a crime will violate the terms of his pretrial probation, even though a growing body of research has shown flaws in such decisions made by machines. An AI program that set school schedules in Boston was scrapped after outcry from working parents and others who objected to its disregard of their schedules.
That’s why, at the M.I.T. Media Lab, we are starting to refer to such technology as “extended intelligence” rather than “artificial intelligence.” The term “extended intelligence” better reflects the expanding relationship between humans and society, on the one hand, and technologies like AI, blockchain, and genetic engineering on the other. Think of it as the principle of bringing society or humans into the loop.
We’re now awakening to the implications that many of these technologies have for individuals and society.
Typically, machines are "trained" by AI engineers using huge amounts of data. Engineers decide what data is used, how it's weighted, the type of learning algorithm used, and a variety of other parameters used to create a model that is accurate and efficient in making decisions and providing accurate insights. The goal is to teach machines how to learn like we do. Facebook’s algorithms, for instance, have observed my activity on the site and figured out that I’m interested in cryptocurrencies and online gaming.
The people training those machines to think are not usually experts in setting pretrial probation terms or planning the schedule of a working parent. Because AI — or more specifically, machine learning — is still very difficult to program, the people training the machines to think are usually experts in coding and engineering. They train the machine using data, and then the trained machine is often tested later by experts in the fields where they will be deployed.
A significant problem is that any biases or errors in the data the engineers used to teach the machine will result in outcomes that reflect those biases. My colleague Joy Buolamwini found, for example, that facial analysis software that classifies gender easily identifies white men, but it has a harder time distinguishing people of color and women — especially women of color.
Another colleague, Karthik Dinakar, is trying to involve a variety of experts in training machines to learn, in order to create what he calls “human-in-the-loop” learning systems. This requires either allowing different types of experts to do the training or creating machines that interact with experts who teach them. At the heart of human-in-the-loop computation is the idea of building models not just from data, but also from the expert perspective on the data.
If an engineer were building algorithms to set terms for pretrial probation, for instance, she might ask a judge to assess the data she’s using. Karthik calls this process of extracting a variety of perspectives “lensing.” He works to fit the “lens” of an expert in a given field into algorithms that can then learn to incorporate that expertise in their models. We believe this has implications for making tools that are both easier for humans to understand and can better reflect relevant factors.
Already, technology and automation are reinforcing and exacerbating social injustice in the name of accuracy, speed, and economic progress.
Iyad Rahwan, a faculty member at the Media Lab, and his group are running a program called “Moral Machines.” Moral Machines uses a website to crowd-source millions of opinions on variants of the “trolley problem,” asking what tradeoffs in public safety might be ethically acceptable in the case of self-driving cars. Some have dismissed such tradeoffs as unlikely or theoretical, but Google filed a patent in 2015 called “Consideration of risks in active sensing for an autonomous vehicle,” which describes how a computer could assign weights, for example, to the risk and cost of a car hitting a pedestrian versus that car getting hit by an oncoming vehicle. In March, a pedestrian was killed by a self-driving car, the first such death recorded.
Kevin Esvelt, a genetic engineer and Media Lab faculty member, won praise for seeking input from residents of Nantucket and Martha’s Vineyard on his ideas for engineering a mouse that would be resistant to Lyme disease. He invited communities to govern the project, including the ability to terminate it at any time. His team would be the “technical hands,” which could mean working on a technology for a decade or more and then not being able to deploy it. That’s a big step for science.
We also need humans in the loop to develop the metrics that will fairly assess the costs and benefits of new technology. We know that many of the metrics we use to measure the success of the economy — for example gross domestic product, rates of unemployment, the rise and fall of the stock market — don’t include external costs to society and the environment. Already, technology and automation are reinforcing and exacerbating social injustice in the name of accuracy, speed, and economic progress.
...more technologists are beginning to realize that their creations have dark sides.
Factories that once employed 300 people can now employ 20 because robots are much more efficient, much less prone to error, and faster at doing work. Some 2 million truck drivers may be wondering when they will be replaced by autonomous vehicles or drones. Emails now offer a menu of potential responses based on the AI in our computers and phones. How long until our inboxes decide to answer without consulting us?
Restoring balance within, between, and among systems will take time and effort, but more technologists are beginning to realize that their creations have dark sides. Elon Musk, Reid Hoffman, Sam Altman, and others are putting money and resources into trying to understand and mitigate the impact of AI. And there are technical ideas being investigated, like ways for civil society to “plug in” to platforms like Facebook and Google to conduct audits and monitor algorithms. Europe’s new General Data Protection Regulation, which becomes enforceable on May 28, will require social platforms to change the way they collect, store, and deploy the data they collect from their customers.
These are small, promising steps, but they are, in essence, efforts to put the genie back in the bottle. We need social advocates, lawyers, artists, philosophers, and other citizens to engage in designing extended intelligence from the outset. That may be the only way to reduce the social costs and increase the benefits of AI as it becomes embedded in our culture.
Joi Ito is director of the MIT Media Lab, coauthor of Whiplash: How to Survive Our Faster Future, and a columnist for WIRED.
This piece is part of a series exploring the impacts of artificial intelligence on civil liberties. The views expressed here do not necessarily reflect the views or positions of the ACLU.
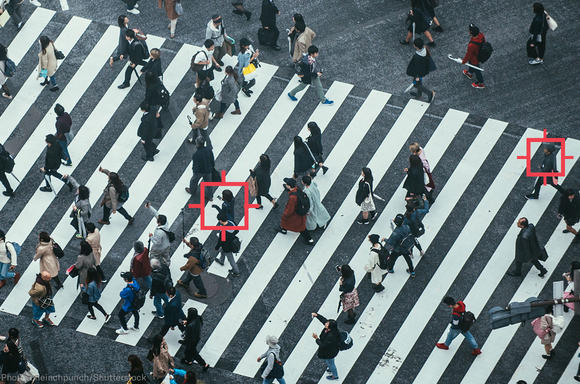
Will Artificial Intelligence Make Us Less Free?
Artificial intelligence is playing a growing role in our lives, in private and public spheres, in ways large and small. Machine learning tools help determine the ads you see on Facebook and routes you take to get to work. They might also be making decisions about your health care and immigration status.
Digital Dystopia: The Danger in Buying What the EdTech Surveillance Industry is Selling
Digital Dystopia: The Danger in Buying What the EdTech Surveillance Industry is Selling, an ACLU research report, examines the EdTech Surveillance...
Source: American Civil Liberties Union
Donate to the ACLU
The ACLU has been at the center of nearly every major civil liberties battle in the U.S. for more than 100 years. This vital work depends on the support of ACLU members in all 50 states and beyond.
We need you with us to keep fighting — donate today.
Contributions to the ACLU are not tax deductible.