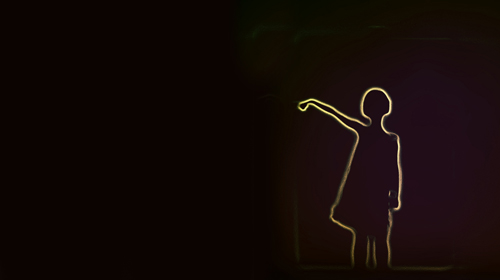
A conversation like this may well take place not far in the future:
Insurance rep: How may I help you?
Man: Yes, hello, I recently received a notice that my insurance has been cancelled, and I wanted to find out why. The letter I got was really vague about it.
The rep verifies his account and identity
Insurance rep: Unfortunately, our Actuarial Benefit Liability Assessment team has terminated your account because of unacceptable risk. They regularly review the risk profile of our customers, and on very rare occasions decide to terminate certain contracts.
Man: Terminate contracts? What information has this decision been based on?
Insurance rep: Those determinations are based on the full range of information about your life and activities that is available to us.
Man: But what was it about my life that you decided made me an “unacceptable risk”?
Insurance rep: I’m afraid I cannot tell you that.
Man: Cannot tell me! I should think I have a right to know that—what if your information is false?
Insurance rep: I’m very sorry, we just can’t tell you that information.
Man: Why on earth NOT! I demand to speak with a manager!
Insurance rep: I mean, we literally cannot tell you what information this decision was based on, because we do not know ourselves.
Think this is far-fetched? The Register ran a piece last year with the somewhat overdramatic title, “Google’s computers OUTWIT their humans.” As the reporter writes, “Google no longer understands how its ‘deep learning’ decision-making computer systems have made themselves so good at recognizing things in photos.” The piece relays a talk by a Google software engineer describing how the company’s software trained to identify certain objects became more effective at doing so than humans looking at pictures. Even the engineer himself could not “explain exactly how the system has learned to spot certain objects,” and had no idea how to give a computer instructions on how to do so.
The opaque characteristic of advanced algorithms is not news to those who have followed how modern nonlinear machine learning systems work, but I find it one of the most fascinating developments in technology: the gradual escape of computers from the confines of clear and understandable logic as they get progressively more complicated. We’re headed toward a world run by more and more computer systems that are increasingly distant from human comprehension.
Supporters of automated law enforcement in various contexts often cite the “objectivity” of algorithms as one of the benefits of this approach. While we quirky and biased humans might be unable to escape having our decisions be shaped by irrational criteria such as race, ethnicity, and gender, a “neutral” algorithm will look at “just the facts.” You bought a one-way ticket, at the last minute, using cash, and are traveling alone? Boom! Extra screening for you. And yay, we can assure you your ethnicity had no role in the decision.
While this benefit of computerized enforcement can be real in some circumstances, there are a number of problems with this view (which we might call "algorithmic positivism"). The blind, discretionless application of simple rules to the infinite variety of circumstances in human life can bring about its own injustices. And no algorithm is neutral and value-free; the variables that a computer is directed to process and how it is directed to weigh them inevitably incorporate all kinds of value judgments.
But as computers continue to move from applying simple, transparent, and logical algorithms toward increasingly complicated, murky, and self-programmed behavior—as they become more intelligent and “human-like”—the full quirkiness and bias of human brains may begin to reassert themselves, in ways that are even less predictable than with humans. (And potentially with less accountability, an angle I wrote about here).
Machine learning will increasingly be applied to the oceans of personal data being collected about us, and in ways that are more opaque and insulated from analysis, challenge, and review. The privacy implications are significant.
As long as the collection of personal data is not limited by privacy reforms, it is all too easy to imagine an insurance company sucking in vast volumes of personal information about individuals’ purchases, communications, movements, health care, and finances—and throwing it into a block box algorithm where results come spitting out according to a logic that is inscrutable to humans. Perhaps the computer detects that soccer-playing 38-year-olds who also love science fiction and French cooking and match 114 other variables happen to be really bad insurance risks.
And to be clear, that computer algorithm may well be correct in the correlations that it finds. Even if those correlations are not fair.
And so we’re going to increasingly have to decide whether we want to surrender some of our control over our own lives (which is the essence of being a citizen of a democracy) to opaque computer algorithms in exchange for the efficiencies that they bring to large institutions, or whether we want to insist that the logic of decisions that affect our lives remain transparent.
Decisions about whether to cede control to computers are a central theme of science fiction (think War Games and Terminator II). But this is one of the first real areas where we will be faced with this question in a big way.